Academic Staff
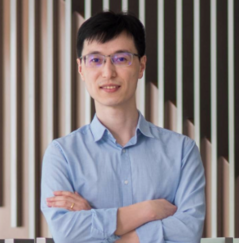
Professor HUANG, Yuanhua 黃淵華 (Joint Appointment with Department of Statistics & Actuarial Science, School of Computing and Data Science)
- PhD (U of Edinburgh)
- BEng (Tsinghua U)
- Assistant Professor
- Bioinformatics
- Machine learning
- Single-cell genomics
- Spatial transcriptomics
- Biomedical artificial intelligence
Dr Huang is an assistant professor in the School of Biomedical Sciences and the Department of Statistics and Actuarial Science at the University of Hong Kong (HKU). Before joining HKU, he was an EBPOD research fellow at the University of Cambridge and the European Bioinformatics Institute (EMBL-EBI). Dr Huang completed his BEng in Automation from Tsinghua University (2013) and PhD in Informatics (Machine learning and computational biology) from the University of Edinburgh (2017). His research lies at the interface between machine learning, cellular genomics, and biomedicine by developing statistical & intelligence methods to decode complex cellular systems in development and diseases.
- Department of Statistics and Actuarial Science, University of Hong Kong
- School of Computing and Data Science, University of Hong Kong
We are a 100% dry lab, developing computational methods to achieve accurate quantifications from noisy and/or sparse sequencing data and discover biological patterns from high dimensional omics data.
We have been working on single-cell data science since the establishment of the lab, focusing on developing statistical machine learning methods to dissect the temporal transitions and spatial interaction among single cells, including RNA velocity methods to reveal cell differentiation trajectory, somatic mutation detection to identify tumor clonal structure, and ligand-receptor interaction to study cell-cell communications. Currently, we further focus “AI for cellular biomedicine” including but not limited to the following topics:
1. Multimodal AI decoding tumor-immune interaction by fusing spatial omics & histological images;
2. Modelling cancer evolution from multi-type mutations using single-cell and spatial omics;
3. Interpretable AI dissecting complex regulation and genetic effects in human development and diseases with single-cell omics;
4. Modelling RNA processing and its intrinsic dynamics, e.g., with RNA velocity, to decipher cell differentiation trajectory and its regulation.
We welcome students to join us to work on existing research directions or related new topics. Please email Dr. Huang (yuanhua@hku.hk) directly for project details and opportunities.
- Huang R. †, Huang X. †, Tong Y., Yan Y.N., Leung S.Y., Stegle O. and Huang Y.# “Robust analysis of allele-specific copy number alterations from scRNA-seq data with XClone.” Nature Communications, 2024, 15 (1), 6684.
- Qiao C. and Huang Y.# “Reliable imputation of spatial transcriptomes with uncertainty estimation and spatial regularization.” Patterns, 2024, 5: 101021.
- Li Z., Wang T., Liu P.# and Huang Y.# “SpatialDM for rapid identification of spatially co-expressed ligand–receptor and revealing cell–cell communication patterns.” Nature Communications, 2023, 14: 3995.
- Hou R., Hon C.C., and Huang Y. # “CamoTSS: analysis of alternative transcription start sites for cellular phenotypes and regulatory patterns from 5’ scRNA-seq data.” Nature Communications, 2023, 14:7240.
- Gao M., Qiao C., and Huang Y.# “UniTVelo: temporally unified RNA velocity reinforces single-cell trajectory inference.” Nature Communications, 2022, 13, 6586.
- Kwok, A. W. C., Qiao, C., Huang, R., Sham, M. H., Ho, J. W.#, & Huang, Y.# “MQuad enables clonal substructure discovery using single cell mitochondrial variants.” Nature communications, 2022, 13(1): 1-10.
- Hou, R., & Huang, Y.# “Genomic sequences and RNA binding proteins predict RNA splicing efficiency in various single-cell contexts.” Bioinformatics. 2022, btac321.
- Qiao, C., & Huang, Y.# “Representation learning of RNA velocity reveals robust cell transitions.” Proceedings of the National Academy of Sciences, 2021, 118(49).
- Huang, X., & Huang, Y.#. “Cellsnp-lite: an efficient tool for genotyping single cells.” Bioinformatics, 2021, 37(23): 4569-4571.
- Huang, Y.#, & Sanguinetti, G.# “BRIE2: computational identification of splicing phenotypes from single-cell transcriptomic experiments.” Genome biology, 2021, 22(1): 1-15.
- 2022, NSFC Excellent Young Scientists Fund (Hong Kong & Macau)
- 2018, Chinese Government Award for Outstanding Self-Financed Students Abroad
- 2017, EBPOD postdoctoral fellowship, University of Cambridge and EMBL-European Bioinformatics Institute
- 2017, Best poster award, High Throughput Sequencing algorithms (HiTSeq) workshop, ISMB/ECCB Conference
Last update: March 10, 2025